This design involves only two principles i.e., the principle of replication and the principle of randomization of experimental designs.
Formal Experimental Design
Completely
randomized design:
This design involves only two
principles i.e., the principle of replication and the principle of
randomization of experimental designs. Among all other designs this is the
simpler and easier because it’s procedure and analysis are simple. The
important characteristic of this design is that the subjects are randomly
assigned to experimental treatments. For example, if the researcher has 20
subjects and if he wishes to test 10 under treatment A and 10 under treatment
B, the randomization process gives every possible group of 10 subjects selected
from a set of 20 an equal opportunity of being assigned to treatment A and
treatment B. One way analysis of variance (one way ANOVA) is used to analyze
such a design.
Randomized
block design:
R. B. Design is an improvement
over the C.R. design. In the R .B. Design, the principle of local control can
be applied along with the other two principles of experimental designs. In the
R.B. design, subjects are first divided into groups, known as blocks, such that
within each group the subjects are relatively homogenous in respect to some
selected variable. The number of subjects in a given block would be randomly
assigned to each treatment. Blocks are the levels at which we hold the
extraneous factor fixed, so that its contribution to the total variability of
data can be measured. The main feature of the R.B. design is that, in this,
each treatment appears the same number of times in each block. This design is
analyzed by the two-way analysis of variance (two-way ANOVA) technique. Latin
squares design:
The Latin squares design (L.S
design) is an experimental design which is very frequently used in agricultural
research. Since agriculture depends upon nature to a large extent, the
condition of research and investigation in agriculture is different than the
other studies. For example, an experiment has to be made through which the
effects of fertilizers on the yield of a certain crop, say wheat, are to be
judged. In this situation, the varying fertility of the soil in different
blocks in which the experiment has to be performed must be taken into
consideration; otherwise the results obtained may not be very dependable
because the output happens to be the effects of not only of fertilizers, but
also of the effect of fertility of soil. Similarly there may be the impact of
varying seeds of the yield. In order to overcome such difficulties, the L.S.
design is used when there are two major extraneous factors such as the varying
soil fertility and varying seeds. The Latin square design is such that each
fertilizer will appear five times but will be used only once in each row and in
each column of the design. In other words, in this design, the treatment is so
allocated among the plots that no treatment occurs more than once in any one
row or any one column. This experiment can be shown with the help of the
following diagram:
From the above diagram, it is clear that in L.S.
design the field is divided into as many blocks as there are varieties of
fertilizers. Then, each block is again divided into as many parts as there are
varieties of fertilizers in such a way that each of the fertilizer variety is
used in each of the block only once. The analysis of L.S. design is very
similar to the two-way ANOVA technique.
Factorial
design:
Factorial designs are used in
experiments where the effects of varying more than one factor are to be
determined. These designs are used more in economic and social matters where
usually a large number of factors affect a particular problem. Factorial
designs are usually of two types:
1. Simple factorial designs and
2. Complex factorial designs.
Simple
factorial design:
In simple factorial design, the
effects of varying two factors on the dependent variable are considered but
when an experiment is done with more than two factors, complex factorial
designs are used. Simple factorial design is also termed as a ‘two-factor-factorial
design,’ whereas complex factorial design is known as ‘multi-factor-factorial
design.
Complex
factorial designs:
When the experiments with more
than two factors at a time are conducted, it involves the use of complex
factorial designs. A design which considers three or more independent variables
simultaneously is called a complex factorial design. In case of three factors
with one experimental variable, two treatments and two levels, complex factorial
design will contain a total of eight cells. This can be seen through the
following diagram:
A pictorial presentation is given of the design shown
above in the following: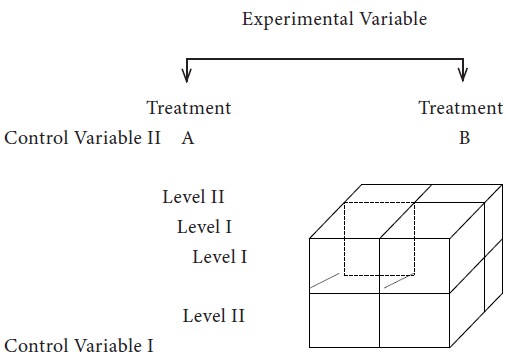
The dotted line cell in this
diagram corresponds to cell I of the above stated 2x2x2 design and is for
treatment A, level I of the control variable 1, and levelIi of the control
variable 2. From this design, it is possible to determine the main effects for
three variables i.e., one experimental and true control variables. The
researcher can also determine the interaction between each possible pair of
variables (such interactions are called ‘first order interactions’) and
interaction between variable taken in triplets (such interactions are called
second order interactions). In case of a 2x2x2 design, the further given first
order interactions are possible:Experimental
variable with control variable 1 | (or EV
x CV 1); |
Experimental
variable with control variable 2 | (or EV
x CV 2); |
Control
variable 1 with control variable 2 | (or CV
1 x CV 2); |
There will be one second order interaction as well
in the given design (it is between all the three variables i.e., EV x CV 1 x CV
2). To determine the main effect for
the experimental variable, the researcher must necessarily compare the combined
mean of data in cells 1, 2, 3 and 4 for Treatment A with the combined mean of
data in cells 5,6,7 and 8 for Treatment B. In this way the main effect of
experimental variable, independent of control variable 1 and variable 2, is
obtained. Similarly, the main effect for control variable 1, independent
experimental variable and control variable 2, is obtained if we compare the
combined mean of data in cells 1, 3, 5 and 7 with the combined mean of data in
cells 2, 4, 6 and 8 of our 2x2x2 factorial design. On similar lines, one can
determine the effect of control variable 2 independent of experimental variable
and control variable 1, if the combined mean of data in cells 1,2,5 and 6 are
compared with the combined mean of data in cells 3,4,7 and 8. To obtain the first order
interaction, say, for EV x CV 1 in the above stated design, the researcher must
necessarily ignore control variable 2 for which purpose he may develop 2x2
design from the 2x2x2 design by combining the data of the relevant cells of the
latter design as has been shown on next page:
Similarly, the researcher can
determine other first order interactions. The analysis of the first order
interaction in the manner described above is essentially a simple factorial
analysis as only two variables are considered at a time and the remaining ones
are ignored. But the analysis of the second order interaction would not ignore
one of the three independent variables in case of a 2x2x2 design. The analysis
would be termed as a complex factorial analysis. It may, however, be remembered
that the complex factorial design need not necessarily be of 2x2x2 type design,
but can be generalized to any number and combinations of experimental and
control independent variables. Of course, the greater the number of independent
variables included in a complex factorial design, the higher the order of the
interaction analysis possible. But the overall task goes on becoming more and
more complicated with the inclusion of more and more independent variables in
our design. Factorial
designs are used mainly because of the two advantages - (i) They provide equivalent accuracy (as happens in the case of experiments
with only one factor) with less labour and as such are source of economy. Using
factorial designs, we can determine the effects of two (in simple factorial design)
or more (in case of complex factorial design) factors (or variables) in one
single experiment. (ii) they permit various other comparisons of interest. For
example, they give information about such effects which cannot be obtained by
treating one single factor at a time. The determination of interaction effects
is possible in case of factorial designs.
Tags : Research Methodology - Experiments
Last 30 days 7068 views