As a part of investigation, samples are drawn from the population and results are derived to help in taking the decisions.
Testing
of hypothesis:
As a part of investigation,
samples are drawn from the population and results are derived to help in taking
the decisions. But such decisions involve an element of uncertainty causing
wrong decisions. Hypothesis is an assumption which may or may not be true about
a population parameter. For
example, if we toss a coin 200 times, we may get 110 heads and 90 tails. At this instance, we are interested in testing
whether the coin is unbiased or not.
Therefore, we may conduct a test
to judge the significance of the difference of sampling or otherwise. To carry
out a test of significance, the following procedure has to be followed:
1.
Framing the Hypothesis:
To verify the assumption, which
is based on sample study, we collect data and find out the difference between
the sample value and the population value. If there is no difference found or
the difference is very small then the hypothetical value is correct. Generally
two hypotheses complementary to each offer are constructed, and if one is found
correct, the other is rejected.
(a) Null Hypothesis:
The random selection of the
samples from the given population makes the tests of significance valid for us.
For applying any test of significance we first set up a hypothesis- a definite
statement about the population parameter/s. Such a statistical hypothesis,
which is under test, is usually a hypothesis of no difference and hence is
called null hypothesis. It is usually denoted by Ho. In the words of Prof.
R.A.Fisher “Null Hypothesis is the hypothesis which is tested for possible
rejection under the assumption that
it is true.”
(b) Alternative Hypothesis.
Any hypothesis which is
complementary to the null hypothesis is called an alternative hypothesis. It is
usually denoted by H1. It is very important to explicitly state the alternative hypothesis in
respect of any null hypothesis H0 because the acceptance or rejection of Ho is meaningful only if it is
being tested against an opposite hypothesis. For example, if we want to test
the null hypothesis that the population has a specified mean µ0(say), i.e., H0:µ=µ then the alternative hypothesis could be:
(i) H1:µ≠µ0 (i.e., µ>µ0 or µ<µ0)
(ii) H1: µ>µ0 (iii) H1: µ<µ0
The alternative hypothesis (i) is
known as a two-tailed alternative and the alternatives in (ii) and (iii) are
known as right-tailed and left-tailed alternatives. Accordingly, the
corresponding tests of significance are called two-tailed, right-tailed and
left-tailed tests respectively.
The null hypothesis consists of
only a single parameter value and is usually simple while alternative
hypothesis is usually composite.
Types Of
Errors In Testing Of Hypothesis:
As stated earlier, the inductive
inference consists in arriving at a decision to accept or reject a null
hypothesis (Ho) after inspecting only a sample from it. As such an element of
risk – the risk of taking wrong decision is involved. In any test procedure,
the four possible mutually disjoint and exhaustive decisions are:
1. Reject Ho
when actually it is not true i.e., when Ho is false.
2. Accept Ho
when it is true.
3. Reject Ho
when it is true.
4. Accept Ho
when it is false.
The
decisions in (i) and (ii) are correct decisions while the decisions in (iii)
and (iv) are wrong decisions. These decisions may be expressed in the following
dichotomous table:
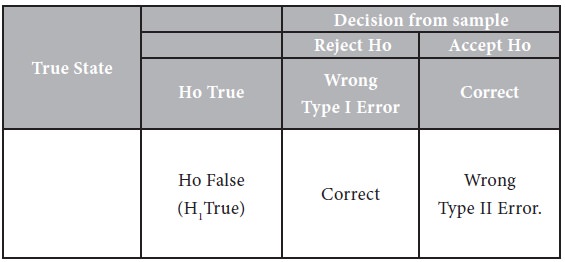
Thus, in testing of hypothesis we are likely to commit
two types of errors. The error of rejecting Ho when Ho is true is known as Type
I Error and the error of accepting Ho when Ho is false is known as Type II
Error.
For example, in the Industrial Quality Control, while
inspecting the quality of a manufactured lot, the Inspector commits Type I
Error when he rejects a good lot and he commits Type II Error when he accepts a
bad lot.
Tags : Research Methodology - Questionnaire & Sampling
Last 30 days 810 views